Generative AI
October 27, 2024· Amol Walunj
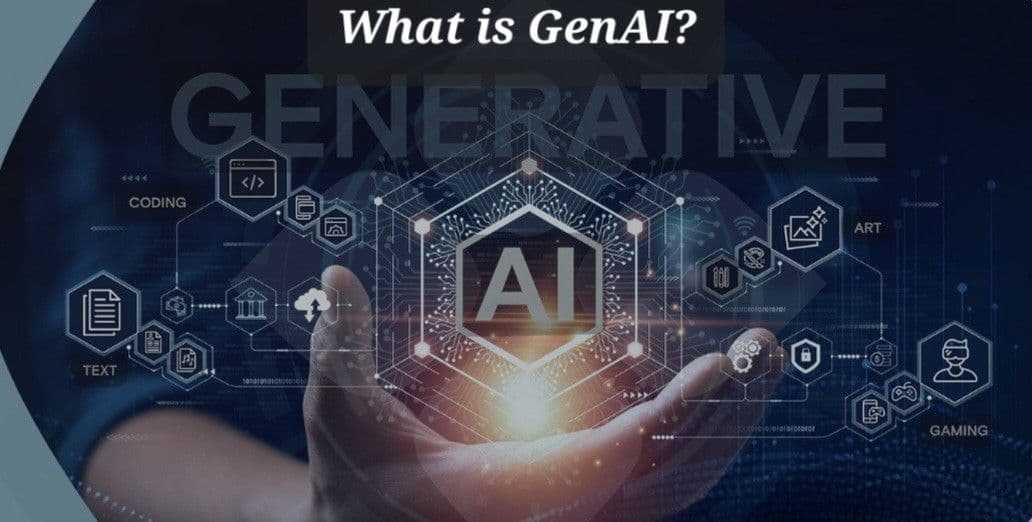
As technology continues to evolve, Generative AI has emerged as a powerful tool reshaping industries from healthcare to marketing. By producing data similar to its training data—whether it’s text, images, or even code—Generative AI is transforming business processes, enhancing creativity, and unlocking unprecedented levels of efficiency. Here, we delve into the applications, technologies, and trends driving Generative AI’s success and shaping its future.
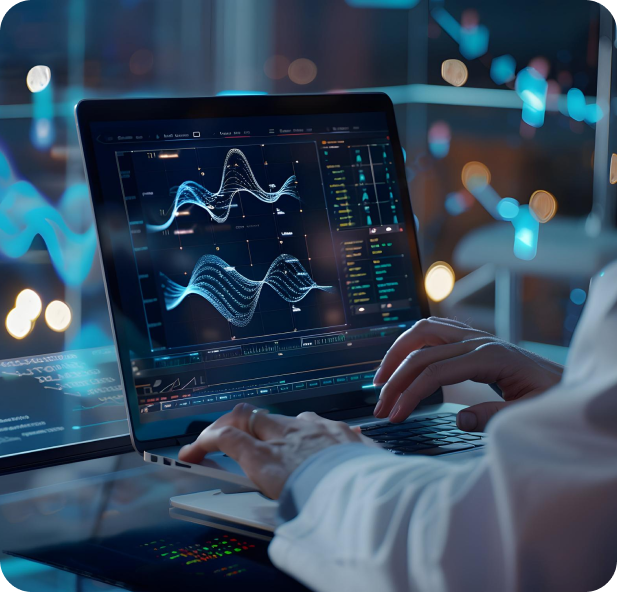
What is Generative AI?
Generative AI refers to systems that create new, unique data that mimics the patterns and characteristics of the original training data. Through advanced algorithms, especially those built on neural networks, it generates outputs in response to specific inputs. Unlike traditional AI, which focuses on classification or prediction, Generative AI models are capable of producing novel content, making them ideal for creative tasks, data augmentation, and simulation.
Key Use Cases of Generative AI Across Industries
1. Content Creation and Personalization in Media and Marketing
Generative AI has become indispensable in content creation, from writing blog posts and social media captions to generating visually engaging advertisements. For marketers, tools like ChatGPT and DALL-E create text and image content at scale, which improves productivity while maintaining brand voice and consistency. AI also enables personalized marketing by generating content based on individual consumer profiles and preferences, enhancing engagement and conversion rates
2. Healthcare Innovation
In healthcare, Generative AI powers advancements in diagnostics, drug discovery, and synthetic data creation. Researchers leverage AI-generated synthetic data to conduct simulations and model diseases in a privacy-compliant manner, a significant advancement in fields where patient data is restricted. Generative models are also used in predicting molecular structures, accelerating drug discovery by identifying potential compounds and simulating their interactions
3. Finance and Algorithmic Trading
Financial institutions are using Generative AI for everything from fraud detection to high-speed algorithmic trading. By analyzing large datasets in real-time, AI systems identify patterns and detect unusual transactions, improving fraud prevention. In trading, AI-driven algorithms analyze market conditions and execute trades, often within seconds, to optimize returns and minimize risks. Generative AI also enhances customer service through AI-powered chatbots, which handle routine inquiries and enable personalized financial recommendations
4. Design and Manufacturing
Generative AI supports design and engineering by generating novel solutions in architecture, industrial design, and product development. Engineers use generative models to create optimized structures that maximize strength while minimizing material use, resulting in cost-effective and sustainable designs. For instance, automotive and aerospace companies apply AI to produce lightweight yet durable components, enhancing both performance and sustainability
5. Gaming and Virtual Reality (VR)
Generative AI enriches gaming and VR by generating adaptive game environments, levels, and storylines. This adds an interactive and personalized element to games, allowing players to experience unique journeys each time they play. AI also enables real-time character responses and environment changes, contributing to more immersive experiences in VR applications
Core Technologies Driving Generative AI
- Transformers:
The transformer architecture, introduced in models like GPT and BERT, relies on attention mechanisms to handle large amounts of data, enabling nuanced understanding and generation of text. These models have revolutionized natural language processing (NLP) by improving the quality and coherence of generated responses
- Generative Adversarial Networks (GANs):
GANs consist of two neural networks—a generator and a discriminator—that compete to produce increasingly realistic data. They are widely used in image generation, such as creating lifelike visuals, and for applications like deepfake creation. GANs are especially popular in fields requiring high-quality, creative visuals
- Diffusion Models:
Diffusion models generate data by gradually adding and then removing noise, an approach that excels in creating high-quality images. These models are an alternative to GANs and are used in applications like DALL-E and Stable Diffusion, which produce realistic images from text prompts
- Variational Autoencoders (VAEs):
VAEs compress data into a lower-dimensional space and then reconstruct it, a useful feature in applications like 3D modeling and molecular structure generation. They are commonly employed for image generation and data compression, particularly in healthcare and scientific research
Emerging Trends in Generative AI
1. Ethics and AI Safety
With growing accessibility, there is an increased focus on the ethical use of Generative AI. Organizations and researchers are implementing strategies to ensure AI-generated content is fair, unbiased, and compliant with privacy regulations. Establishing ethical guidelines is crucial as the use of Generative AI expands across various sectors
2. Generative AI in the Workplace
As Generative AI tools become more advanced, they are enhancing productivity across industries. Developers, for example, can automate code documentation and refactoring, freeing them to focus on complex problem-solving. Surveys show that AI-driven tools improve developer satisfaction and productivity, which in turn supports employee retention
3. Real-time Applications and Customer Experience
Real-time applications, such as interactive customer service and live transcription, are now feasible with lightweight, optimized AI models. Generative AI improves customer experiences by providing immediate responses and personalized interactions, a trend that is gaining momentum in customer-centric industries like retail, finance, and hospitality
4. Synthetic Data Generation for Privacy
Generative AI’s ability to create synthetic data is invaluable in industries where data privacy is critical. By generating realistic datasets that mirror actual data without compromising privacy, organizations can train models more effectively. Synthetic data is increasingly used in healthcare, finance, and government sectors, where data access is often restricted
5. Increased Investment and Innovation
Major tech companies and venture capitalists are heavily investing in Generative AI. The field is expanding with specialized models designed for specific tasks, such as legal document generation or molecular simulation in pharmaceuticals. With ongoing investments, we can expect continued innovation in model architectures and industry applications
Conclusion
Generative AI is proving to be a transformative technology, driving efficiency, creativity, and new opportunities across sectors. From revolutionizing content creation and healthcare to enhancing financial services and manufacturing, its applications are vast and impactful. As the technology advances, we will continue to see developments in ethical AI, real-time applications, and the use of synthetic data, all of which will contribute to an increasingly AI-powered future.
By understanding the core technologies, staying informed on industry trends, and responsibly implementing Generative AI, organizations can harness this innovation to drive value, streamline operations, and maintain a competitive edge.
At Cogninest AI, we specialize in helping companies build cutting-edge AI solutions. To explore how we can assist your business, feel free to reach out to us at sales@cogninest.ai